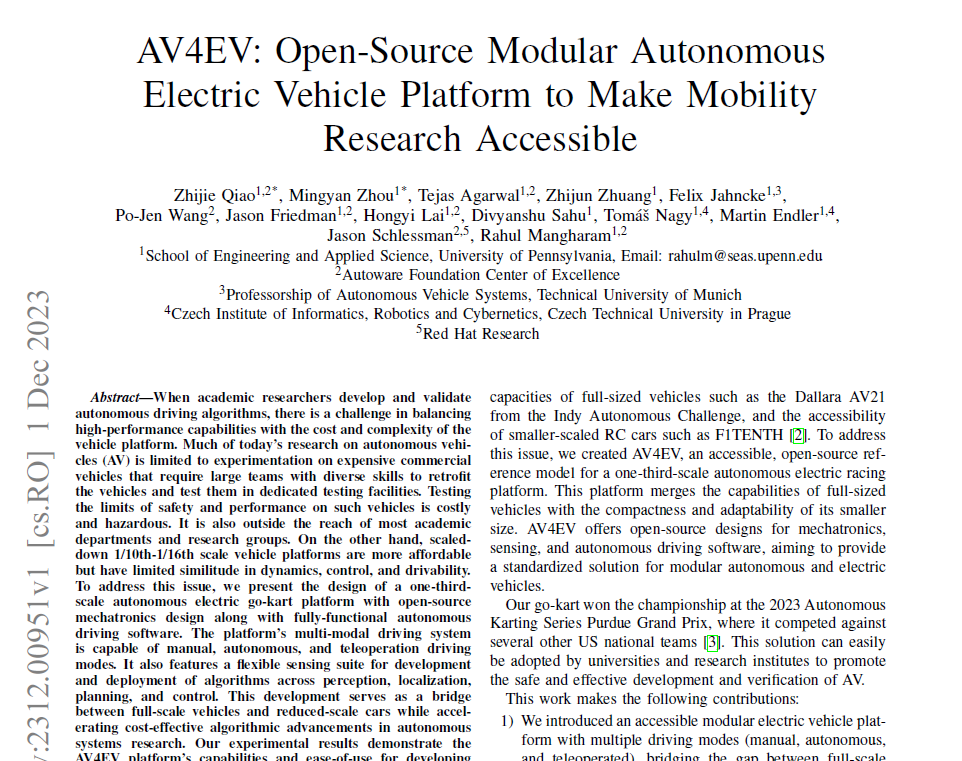
Abstract
When academic researchers develop and validate autonomous driving algorithms, there
is a challenge in balancing high-performance capabilities with the cost and complexity of the
vehicle platform. Much of today’s research on autonomous vehicles (AV) is limited to
experimentation on expensive commercial vehicles that require large teams with diverse
skills to retrofit the vehicles and test them in dedicated testing facilities. Testing
the limits of safety and performance on such vehicles is costly and hazardous. It is
also outside the reach of most academic departments and research groups. On the other
hand, scaled- down 1/10th-1/16th scale vehicle platforms are more affordable but have
limited similitude in dynamics, control, and drivability. To address this issue, we present
the design of a one-third- scale autonomous electric go-kart platform with open-source
mechatronics design along with fully-functional autonomous driving software. The
platform’s multi-modal driving system is capable of manual, autonomous, and teleoperation
driving modes. It also features a flexible sensing suite for development and deployment
of algorithms across perception, localization, planning, and control. This development
serves as a bridge between full-scale vehicles and reduced-scale cars while accelerating cost-effective algorithmic advancements in autonomous systems research. Our experimental results demonstrate the AV4EV platform’s capabilities and ease-of-use for
developing new AV algorithms. All materials are available at AV4EV.org to stimulate collaborative within AV and electric vehicle (EV) communities.
is a challenge in balancing high-performance capabilities with the cost and complexity of the
vehicle platform. Much of today’s research on autonomous vehicles (AV) is limited to
experimentation on expensive commercial vehicles that require large teams with diverse
skills to retrofit the vehicles and test them in dedicated testing facilities. Testing
the limits of safety and performance on such vehicles is costly and hazardous. It is
also outside the reach of most academic departments and research groups. On the other
hand, scaled- down 1/10th-1/16th scale vehicle platforms are more affordable but have
limited similitude in dynamics, control, and drivability. To address this issue, we present
the design of a one-third- scale autonomous electric go-kart platform with open-source
mechatronics design along with fully-functional autonomous driving software. The
platform’s multi-modal driving system is capable of manual, autonomous, and teleoperation
driving modes. It also features a flexible sensing suite for development and deployment
of algorithms across perception, localization, planning, and control. This development
serves as a bridge between full-scale vehicles and reduced-scale cars while accelerating cost-effective algorithmic advancements in autonomous systems research. Our experimental results demonstrate the AV4EV platform’s capabilities and ease-of-use for
developing new AV algorithms. All materials are available at AV4EV.org to stimulate collaborative within AV and electric vehicle (EV) communities.